PRESS RELEASE
Pack It, Knit It, Link It: Challenges in 3D Printing, Textile Production, and Beyond

Pack It, Knit It, Link It: Challenges in 3D Printing, Textile Production, and Beyond
CHICAGO – June 20, 2023 —The vast field of computer graphics and interactive techniques spans the gamut of industries, from gaming and film to biomedical and manufacturing. The core of SIGGRAPH 2023, the 50th iteration of an annual global — and marquis — conference for the brightest and most inventive minds in the field, is a platform to share and showcase the bold, innovative research and products that are changing — and improving — the way we live, work, and play.
Research expected to debut as part of the conference’s Technical Papers program are “ones to watch” — exciting new technologies, ideas, and algorithms that span all areas of graphics and interactive techniques. Particularly noticeable this year is the emergence of research methods that extend beyond the digital world and address creation of real-life content.
“A lot of research in computer graphics has been about determining the best way to visualize various real-world phenomena using computers, and in doing so, there are often details that are elided to fit such complicated things into the computational framework,” says Jenny Lin, a lead author of one of the featured new research projects that will be showcased at SIGGRAPH 2023. Lin and her collaborators have devised a formal semantics framework for machine knitting programs, applying mathematics to describe anything a knitting machine can make.
“Going from virtual representations to the physical world involves addressing details that we often take for granted when going about our daily lives,” she adds. “There’s a very natural connection between these two directions, and there’s something very gratifying about using the language of computers to understand and improve something as tactile and grounded as knitting.”
This year, computer scientists, artists, developers, and industry experts around the world will convene 6–10 August in Los Angeles for SIGGRAPH 2023. As a preview of the Technical Papers program, here is a sampling of three novel computational methods and their unique approaches to real-world applications.
Equipped to Make It Fit
Additive manufacturing, better known as 3D printing, brings digitally designed products to life. It allows for unprecedented freedom of 3D geometries and it gives manufacturers the ability to produce parts on demand, and locally. With 3D printing, supply chain management can be simplified, and it is easier to transition from engineering iterations to full manufacturing.
3D printing is undergoing a transition from being a prototyping technology to being a manufacturing technology. However, the main roadblock is the overall cost of the manufactured part. 3D printing hardware, materials, and human labor all drive the cost of the technology. The drive for higher cost efficiency requires printing in batches where parts are tightly packed in the 3D printer’s build volume to maximize the number of printed parts per batch. One of the main limitations of this process is the limited utilization of the build volume due to the computational complexity of the packing process./h6>
In a collaboration between MIT and Inkbit, a 3D manufacturer specializing in polymer parts, researchers are addressing the complex problem — and headache — of digitally packing many parts in a single container with multiple constraints. To date, many part models are virtually placed in the printing tray, also referred to as “nesting”, and the printer executes the job printing the whole tray. The problem with this process is that the container isn’t densely packed and there isn’t an efficient method to automate and ensure the 3D printers are printing the maximum volume of parts in a designated container.
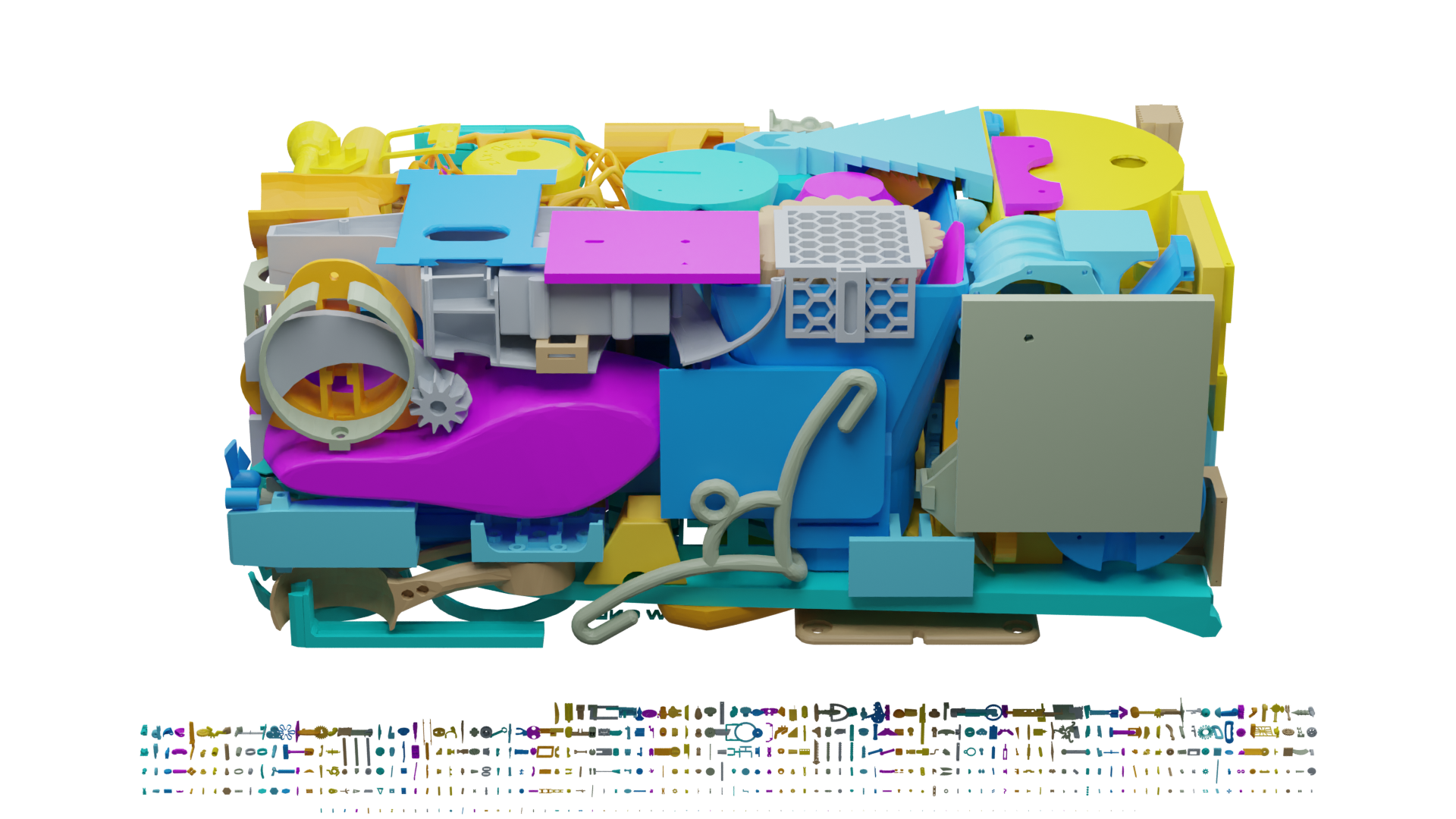
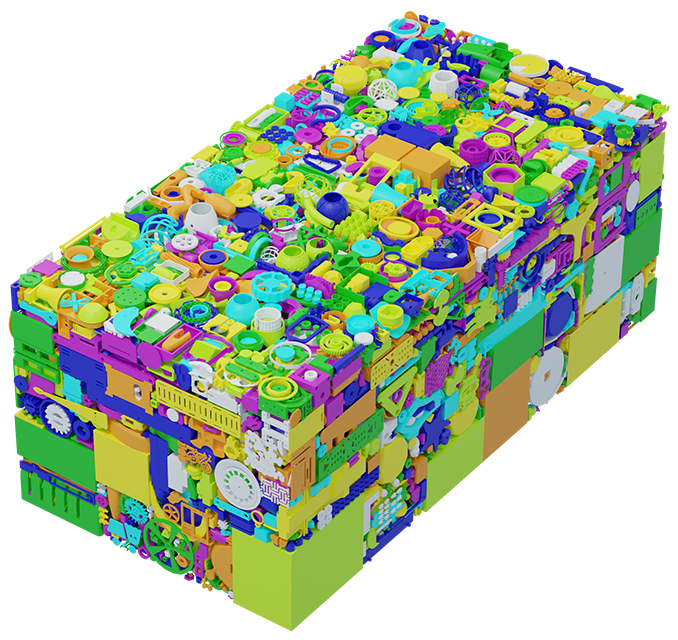